Big data analytics has revolutionized numerous industries, including retail banking. Banks can use big data analytics to better manage risks and minimize losses. Banks use this technology to analyze large volumes of data to spot patterns that enable more informed decision-making. Bahaa Abdul Hussein in this article explores how big data analytics is revolutionizing risk management for retail banks.
Identification of Risks
A key step in mitigating risks is recognizing them early, and big data analytics provides banks with an efficient method for doing just that. Retail banks can now use big data analytics to analyze customer, transaction, and third-party data sources for potential risks they should manage. For instance, identifying customers likely to default on loans, fraudulent transactions, or possible fraudsters among customers or transactions. By recognizing risks early enough and taking steps to mitigate them quickly, they can prevent losses from occurring later down the road.
Real-time Monitoring
Big data analytics facilitate real-time monitoring of transactions, an essential element of risk management. Retail banks can now utilize big data analytics to observe transactions in real-time and detect any irregularities or suspicious activities immediately. This helps identify any potential fraudsters while taking appropriate steps to minimize losses. For example, if an unusually large transaction comes through without warning from one of their customers, banks could flag it as a possible risk and investigate further.
Credit Risk Evaluation
Risk assessment in retail banking is an integral component of risk management. Big data analytics assists banks with this assessment process by examining large volumes of customer and transaction data, credit scores and third-party information compiled from various sources. This allows them to identify any potential risks and make more informed lending decisions – for instance, by identifying customers likely to default and altering lending criteria accordingly.
Customer Segmentation
Big data analytics is revolutionizing retail banking risk management. Banks use customer data analysis to segment customers based on their risk profile and offer products and services accordingly. For instance, low-risk customers may receive reduced interest rates on loans and credit cards. Also, high-risk customers might face more stringent lending criteria and higher interest rates on loans and credit cards.
Fraud Detection
Retail banking institutions face an inherent risk from fraud, but big data analytics has revolutionized how they detect and prevent it. By analyzing large volumes of data from multiple sources, banks can analyze patterns indicative of fraudulent activity. It’s like transactions made from multiple devices with similar IP addresses – early detection can prevent losses while safeguarding customer assets.
Conclusion
Big data analytics is revolutionizing retail banking risk management by helping banks analyze large amounts of data to quickly identify risks that threaten losses. When risks are identified early enough, banks can take appropriate actions to mitigate them and prevent losses. Real-time monitoring, credit risk evaluation, customer segmentation, and fraud detection are some key areas where big data analytics has an enormously positive effect. As more retail banks adopt big data analytics, we should see innovative risk strategies enabling better risk management while increasing bottom-line profits.
The story is written by Bahaa Abdul Hussein and has been published by the editorial board of Fintek Diary. Please stay tuned to www.fintekdiary.com
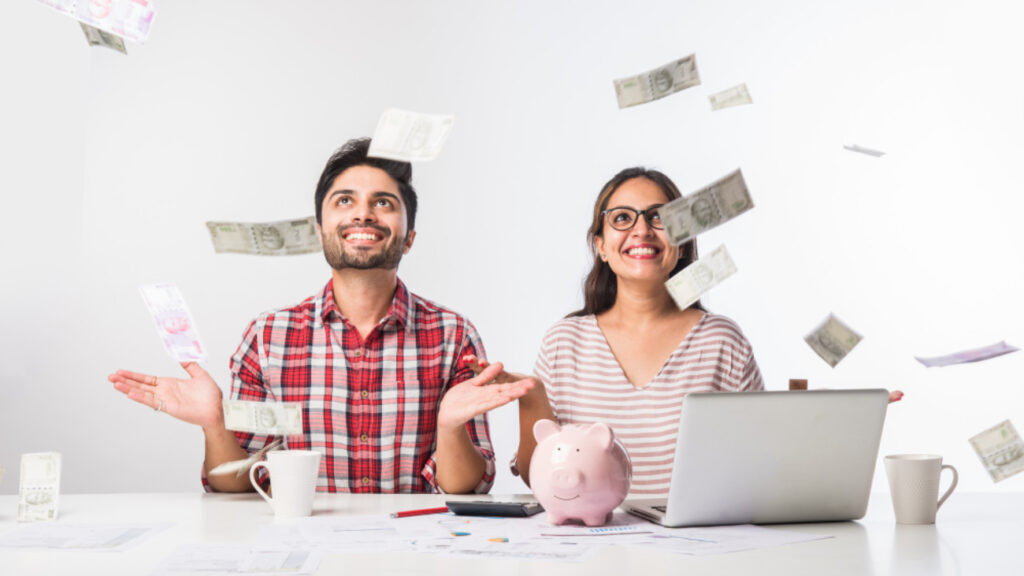